|
Michael Abramowicz, Guest-Blogging:
I'm delighted to welcome George Washington Prof. Michael Abramowicz, who will be guest-blogging about his new book Predictocracy: Market Mechanisms for Private and Public Decision Making, being released this week by the Yale University Press. (Michael and I were briefly colleagues, when he was a lawprof at George Mason, and I was visiting there for a semester.)
Michael's book argues that prediction markets should be widely employed in decision making, because -- when properly designed -- they tend to provide a good algorithm for aggregating different points of view into a single forecast. A decisionmaking institution would be better off using this algorithm than relying on individual decisionmakers to develop their own forecasts, whether explicitly or implicitly.
At its most ambitious, the book defends what Michael calls "normative markets," in which the forecast is of a normative assessment by a decision maker to be randomly selected from a group. Sometimes, he argues, it might be better to rely on a forecast of the decision of a single randomly selected member of a group, rather than on an actual decision of all or a subset of the group members.
Michael will start by addressing some common objections to prediction markets and by outlining their institutional advantages. He'll then offer some of his ideas both for innovative designs and applications of prediction markets. And, finally, he'll explain and defend the broader theory behind normative markets. I'm very much looking forward to seeing Michael's posts.
An Intro to Prediction Markets and the Liquidity Problem:
Thanks, Eugene! I am pleased to be a guest conspirator, and I'm looking forward to writing about Predictocracy. I imagine most readers here are familiar with prediction markets, so I'll start with only a brief explanation of how prediction markets usually work (a longer explanation from my book is here).
In a prediction market, traders can buy and sell contracts that will pay off should a particular event occur. For example, as of this writing, on Intrade.com, you can buy for approximately 58 cents on the dollar a contract that will pay off should Sen. John McCain win the Republican nomination. The current prices at which people are willing to buy and sell McCain shares translate into an estimate that McCain has approximately a 58% chance of being the nominee.
Most of the markets that the book imagines, however, differ in a fundamental way from the Intrade markets. While Intrade charges for its services, the markets that Predictocracy envisions would generally be subsidized by institutions (such as businesses or governments) willing to pay for the estimates that prediction markets produce.
Appropriately administered subsidies would respond to a common criticism of prediction markets: that on non-sexy topics, prediction markets have too little liquidity to be of much use. Even some Intrade markets attract too little attention, at least some of the time, to be useful; consider the current estimate that Imran Kahn has somewhere between a 10% and a 90% chance of being elected Prime Minister of Pakistan.
A subsidy can solve this problem by rewarding individuals who place aggressive offers to buy and sell contracts. This approach, which I describe here, helps reduce the "bid-ask spread," and thus indirectly increases the incentives of people to develop information and analysis that they might then be able to trade on. An alternative approach, such as Robin Hanson's "market scoring rule," is to create an automated market maker that is willing to buy or sell shares at prices based on the current prediction.
Prediction Markets vs. Conventional Wisdom:
I promised to start by addressing some common criticisms of prediction markets. What better way to start than by attacking my friend, GW colleague, and now co-conspirator Orin Kerr? Orin has at least twice (in 2005, and earlier this month) endorsed the criticism that the election markets don't seem to do much more than track the conventional wisdom. Orin is in good if unfamiliar company; Paul Krugman recently made a similar criticism.
Unfortunately for my attack, I don't entirely disagree. On issues for which there is likely to be lots of public information but little private information, prediction markets reflect what highly informed people believe. No better, but no worse. If you want to know what the probability is that X will be President, you probably won't be surprised by the prediction markets, but on average over many independent events the market's predictions will probably be at least slightly better than the ones you would make on your own.
A stronger version of this criticism insists that the markets are worse than the highly informed conventional wisdom. Critics will say that the markets put too much weight on the pro-Obama pre-New Hampshire polls or the pro-Kerry 2004 exit polls. I'm skeptical of this criticism. At any time, the markets may be slightly off, but if they have obvious, large imperfections, people will trade back to more sensible values.
Usually, such criticisms are made after the fact, and they often reflect hindsight bias. It seems obvious now that election observers put too much weight on the pro-Kerry and pro-Obama polls. But even the most sophisticated analysts may have trouble afterward figuring out why (see here on 2004 and here on 2008).
The real problem is that our models of voter behavior aren't as good as we'd like to think. No one cries foul because Tradesports.com gave the Giants a 1% chance in early October of winning the Super Bowl. Football is full of surprises. But whenever something unexpected happens in an election, we feel that we should have expected it all along.
Some might still object that if prediction markets do no more than reflect the fallible informed conventional wisdom, they aren't worth much. And indeed, election markets may do little but save some of us the time of reading the election news. But in a world of ideology, special interests, and agency costs, institutions could do a lot worse than rely on prediction markets in their decision making. The central argument for prediction markets for me is not that they are magically accurate, but that they are fairly objective.
But there is one other final point, hearkening back to yesterday's post: Many prediction markets that would be useful to institutions are on topics on which there may be little public or private information. It is especially important to constrain opportunistic decisionmakers when they are making claims that few if any people have the information to assess. With subsidies and automated market makers, these markets will not merely reflect existing conventional wisdom, but give incentives to a few individuals to do research and make disinterested forecasts.
Manipulation of Prediction Markets:
The most important objection to governmental use of prediction markets is the danger that third parties might manipulate them. If officials deciding whether to expand a highway use a prediction market to forecast traffic in 20 years, road builders might be willing to lose money on the market if in doing so they can change the forecast and influence the public policy debate.
A partial answer is that the stakes of prediction markets (even the heavily subsidized ones I fantasize about) are sufficiently low that transparent attempts at manipulation are unlikely to have much effect. If George Soros announced that he would be willing to risk up to $1 billion to prop up the share of the Democratic candidate on Intrade, hedge funds would gladly take the other side of unjustifiable offers. Maybe arbitrage can't pop a widely recognized stock market or housing bubble, but arbitrage should succeed in individual prediction markets.
The bigger problem is the possibility of hidden attempts at manipulation. If X is bidding up the traffic forecast contract, this may reflect a genuine subjective probability estimate. If so, everyone else should rationally adjust their estimates in the direction of X's trading, especially since X appears confident. Traders will assign some weight to this possibility, and so will not try to move prices all the way back. If X really is manipulating, X will be at least partly successful.
Note, though, that the reason for X's success is that disinterested traders find trades generally to be informative. If I am playing poker, and think that another player has a tell, I might rationally take this into account. Sometimes, the tell is a fake, but I'm better off looking for tells and taking them into account than wearing a blindfold.
Similarly, given a choice of restricting a prediction market to trusted non-manipulators(e.g., government officials), and leaving it open to all, the open market will tend to produce better information, even though manipulation will sometimes be successful. We can improve performance by identifying traders, especially if some earn reputations for accuracy over time.
Nonetheless, if you're unconvinced, or if you think that manipulation might undermine confidence in government, that's no reason to abandon prediction markets altogether. Instead, one can still use them with a small group of trusted players (whether with real or play money). This is still likely to be better than letting just one of these people make a forecast or averaging all of these officials' forecasts.
For a more complete discussion of this issue in Predictocracy, see here. Also, see this article on a model and this article on an experiment showing that manipulators can increase price accuracy by providing extra market liquidity.
Deliberating with Prediction Markets:
Prediction markets may seem inadequately deliberative. On the election markets, for example, participants trade, but do not ordinarily explain their trades. Decision makers in deliberative bodies, in contrast, seek to persuade one another.
Group deliberation, however, has its own perils, including the danger that polarization will move a group to extremes, as Cass Sunstein has shown. Sunstein argues in Infotopia that prediction markets might therefore be superior in some contexts to deliberation. A recent study shows better forecasts with prediction markets than with group deliberation.
In some contexts, though, prediction markets might be more useful yet if individual participants explained their forecasts. I've proposed a type of prediction market called a deliberative market that can increase incentives that participants have to release information supporting their views. In the deliberative market (see my original paper here and this section of my book), a participant's profit or loss is determined by the market forecast some time after the participant's initial prediction, so a participant can earn money only to the extent that others are persuaded in that time frame.
In a post yesterday on the Overcoming Bias blog, Robin Hanson criticizes my argument for not including a robust enough economic model and for allegedly making unrealistic assumptions. In a reply, I maintain that the point is pretty simple, and the math I used was ample to make it. In the comments to my reply, Robin and I come closer to agreeing about the underlying issue of whether the deliberative market increases incentives for information release.
Chris Hibbert, who has developed the robust Zocalo open source prediction market software, meanwhile, makes the sound point that a possible disadvantage of the deliberative approach is that it may stop individuals who are confident of their views but don't think they can persuade others in the time frame from participating in the market. Sometimes, it might be useful to have both a standard and a deliberative prediction market for the same forecasting problem.
There may be other ways of making prediction markets more transparent. An admittedly more speculative section of the book imagines the "market web," which can be used to break down problems. For example, an election market might include a node forecasting the possibility of a recession. Changes in this node's value would automatically affect the value of other nodes, including ultimately the probability that particular candidates would win the election. Such a web could become complicated very quickly, but it could allow a group to produce a consensus model of a complex phenomenon.
Predicting Decisions and Their Effects:
So far, my posts have implicitly assumed independence between forecasts and decisions. Now, let's consider some ways in which we might structure prediction markets to forecast the decisions themselves and their consequences, so that the forecasts might influence the decisions.
(1) Markets predicting decisions. A market that predicts a decision might end up affecting the decision. Suppose that Eugene is elected dictator, but because of his blogging responsibilities, His Tremendousness must make many decisions. So, he establishes prediction markets forecasting what decisions he will make.
Now, Eugene is presented with a decision to make, and he quickly analyzes the problem and leans toward Decision A. But then he checks the market and sees that it forecasts that he will make Decision B. He wonders, why is that? He looks more carefully and realizes that he has missed some aspects of the problem.
Some of the dynamics of the deliberative market are present here. A trader predicting a decision can profit by developing arguments that will persuade the decision maker. For example, the trader can write an argument for Decision A and bet on Decision A just before releasing the argument. Eugene might thus create a market predicting his decisions as a way of generating research and arguments relevant to those decisions.
(2) Conditional markets. A conditional market predicts some variable contingent on a condition. A simple way to run such a market is to stipulate that all money spent on the prediction market will be refunded if the condition does not occur. For example, one market could predict a corporation's stock price if a corporation decides to build a factory, and a separate market could predict the stock price if it doesn't build the factory. The corporation can compare the forecasts to assess the market's perception of the effect of building the factory on stock price.
These are a useful tool, but there are important caveats. First, small deviations between two markets can't be taken too seriously. If Market A predicts a stock price of $30.00 and Market B predicts a stock price of $30.01, the difference could just be noise. Relatedly, if the condition will have little effect on the stock price, even subsidized prediction markets will give people little incentive to study the effect of the condition. Instead, the subsidy will just give general incentives to study all factors that might affect future stock price.
Second, traders will recognize that information unknown to them may affect the decision. For example, last May, Hillary Clinton's chance of winning the Presidency conditional on being nominated was estimated based on prediction markets at over 70%. That could indicate that Clinton was a strong candidate. It also could mean that the Democrats would stick with a weak candidate like Clinton only if other factors, like the economy, were pointing so strongly in the Democrats' direction that Democratic primary voters did not care about electability.
In our next installment, I'll show that "normative markets" combine the two market approaches considered above.
Normative Prediction Markets:
Suppose that you are a member of a large group that has a large number of decisions to make. It might seem that you have two basic choices.
First, allow everyone to vote on every decision. This approach produces high representativeness (at least if everyone votes), but the votes will be based on little information. Second, allow a subset of the group to make each decision. This approach reduces representativeness, but allows for more informed decision makers.
Democratic institutions combine these two basic approaches in elaborate ways to overcome the trade-off between unrepresentative and uninformed decision making. All enfranchised citizens select a few citizens to serve as legislators, for example, and legislators divide into committees. For different types of decisions, we accept different trade-offs. Three-judge panels are unrepresentative but informed, so in theory we allow them to resolve legal questions but not to change national policy.
None of these solutions is perfect, and we face the usual perils of republican decision making: ignorant voters, special interests, legislative inertia, activist judges, and executive policies highly sensitive to the quadrennial preferences of a small number of voters in places like Florida and Ohio. But we may well structure voting regimes reasonably efficiently given the fundamental trade-off.
There is, however, a way of overcoming this basic trade-off using prediction markets rather than votes. We can commit to selecting someone at random from our group, or from a subset of it, to say what the decision should be. We will require this person to listen to detailed arguments and to produce a detailed explanation. But this will not be our decision. Instead, our decision will be based on the forecast generated by a "normative prediction market" predicting what this person will conclude is best.
Moreover, we don't even need to have someone conduct this evaluation for every decision. We can use a pseudo-random number generator to pick only, say, one-tenth of the decisions for ex post evaluation. Before we make the random selection, we run a conditional normative market, where the condition is that the decision is selected for ex post evaluation. But every time, it is the market's prediction that we will use as the decision.
A summary of the steps: (1) Subsidized conditional market predicts decision. (2) This prediction determines the group's actual decision. (3) Random number from 0 to 1 is drawn; if it's greater than 0.10, all money from market is returned. (4) Person is picked at random from group, and must eventually announce what he or she would have decided. (5) This evaluation is used to determine payouts in the conditional market.
This is a radically new way of making decisions, and I emphasize in the book that there are strong reasons not to transform radically our democratic institutions. I use dramatic examples (e.g., prediction market legislatures, trial by market) to illustrate the approach colorfully, but I don't believe we should rewrite the Constitution. Normative markets could serve as useful inputs into more traditional decision making (change step 2 above to "This prediction provides a recommended decision."), or be used in private settings.
All I want to show here is that this approach, which could also be used in private settings, helps overcome the trade-off between unrepresentative and uninformed decision making.
If the prediction market is sufficiently subsidized, then the prediction can be highly informed. Since we only have a few decisions that need ex post evaluation, and only one decision maker per decision, we can demand a lot of the ex post decision maker, who will then become informed too. Picking a random citizen might not be the best strategy, since an informed dolt is still a dolt, so we might have the ex post evaluator randomly drawn from a body akin to a judiciary (experts selected by indirectly elected representatives).
Meanwhile, the system provides a virtual representativeness. Traders don't know who the actual ex post decision maker would be, so they will average the anticipated decisions of a broad ideological range of potential decision makers. We may be able to increase representativeness still further by delaying decisions a decade or so, so it won't matter if we happen to have an unbalanced set of ex post decision makers at any one time.
Critically, it doesn't matter if the actual ex post decision maker makes a foolish or unrepresentative decision. What matters is the average expected decision, because it is only the prediction of the ex post evaluation that determines policy.
Of course, my claims here depend on my earlier claims that prediction markets will be sufficiently accurate and deliberative.
Nineteenth-century prediction markets:
Those who are interested in Mike Abramowicz's prediction markets posts may also be interested in this new NBER working paper I just saw on SSRN:
Historians have long wondered whether the Southern Confederacy had a realistic chance at winning the American Civil War. We provide some quantitative evidence on this question by introducing a new methodology for estimating the probability of winning a civil war or revolution based on decisions in financial markets. Using a unique dataset of Confederate gold bonds in Amsterdam, we apply this methodology to estimate the probability of a Southern victory from the summer of 1863 until the end of the war.
Our results suggest that European investors gave the Confederacy approximately a 42 percent chance of victory prior to the battle of Gettysburg/Vicksburg. News of the severity of the two rebel defeats led to a sell-off in Confederate bonds. By the end of 1863, the probability of a Southern victory fell to about 15 percent. Confederate victory prospects generally decreased for the remainder of the war.
The analysis also suggests that McClellan's possible election as U.S. President on a peace party platform as well as Confederate military victories in 1864 did little to reverse the market's assessment that the South would probably lose the Civil War.
This paper is also available directly on the NBER site, though I'm not sure whether the general public has free access to it.
Why Normative Markets?
In my last post, I described and gave a general argument for normative prediction markets. If a prediction market forecasts an evaluation by someone to be selected randomly from a body of very educated people (somewhat analogous to the federal judiciary, though perhaps selected in a way that makes it more representative), it will be an informed forecast of an informed decision, and the uncertainty about who the eventual decision maker will be provides for a kind of virtual representativeness.
Now, I'll describe several advantages of normative markets that follow:
(1) More consistent, predictable decision making. The virtual representativeness reduces the danger of idiosyncratic decision making. Of course, there will be some decisions that fall close to the line, but we avoid some situations where it's clear that 2/3 of decision makers would make one decision, but it happens to be someone in the 1/3 of decision makers who gets the final call.
If we can have more consistent, predictable decision making, we also may see a general shift from legal rules to standards. A powerful argument for rules over standards is that only rules can produce consistent and predictable decision making. With normative markets deciding whether legal provisions are followed, standards become relatively more attractive.
(2) More principled decisions. Suppose there is some higher order principle X that the group has precommitted to in advance. Now, we have to make a decision about whether something that the group has decided to do, Y, would be consistent with that high-level principle.
With conventional decision making, the decision maker may well sacrifice X for Y. X may be more important to a decision maker than Y, but a disingenuous argument that Y is consistent with X makes it only slightly less likely that X will be followed in the future. Those who have read Mistretta v. United States should understand what I am talking about.
This is less likely with normative markets, because the evaluation of whether Y is consistent with X will not actually affect whether the group can do Y. That decision has already been made. So, a precommitment to using normative markets can help improve the chance that the group will follow through on its substantive precommitments.
(3) More insulated decisions. It should be harder for a special interest group to influence decision making with normative markets. (Assume for the sake of argument that special interests make decision making worse rather than better.) The judiciary is relatively immune from special interests, and so too could be the pool of ex post evaluators.
A special interest group could try to affect the pool of ex post evaluators, but with many evaluators, each making only a small number of randomly selected decisions on a large number of potential topics, this won't be easy. Moreover, bribing the ex post evaluator would not be enough; the special interest group would have to commit credibly to bribing the evaluator, because the actual ex post decision would not matter.
(4) More scalable decisions. We can easily change the probability that a case is submitted to an ex post evaluator. More decisions would require more subsidies, but we don't have to hire and select more decision makers. Market participation should grow in proportion to subsidies.
Consider, for example, immigration review. From one perspective, this might seem to be one of the worst contexts for prediction markets, because they seem impersonal. But our current system of immigration may be inhumane and capricious. Normative markets could at least eliminate backlogs, in addition to providing more consistent decision making.
Predictocracy vs. Futarchy:
In describing normative markets in my book, I outline the possibility of prediction market-based legislative, judicial, and even executive power, but only for heuristic value. Nonetheless, it is fun to indulge in political science fiction and imagine a government run by prediction markets. I hope that this exercise can convince people that prediction markets are a powerful and flexible tool that may be useful in more modest but still exciting ways.
A predictocracy, then, is a government in which normative markets make the full range of government decisions, except when the prediction market mechanism results in a decision to delegate a decision to some other mechanism (whether traditional or using prediction markets in some other way).
I am not the first to imagine prediction markets serving at the center of government. Robin Hanson has previously defended a form of government that he calls "futarchy." His vision is that the legislature would be limited to defining some objective function (a GDP+ that includes GDP, but also anything else of value). Only policies that conditional markets predict would increase GDP+ would be enacted.
The slight disagreement between Hanson and me may sound to skeptics and even many prediction market enthusiasts like an argument between religious fanatics who have already disengaged from reality. But in Predictocracy, I explain why I prefer predictocracy to futarchy, and Hanson has now respectfully joined the argument.
My principal reasons for preferring predictocracy stem from the caveats that I previously offered about conditional markets. I worry that there will be too much noise in estimating GDP+ to make reliance on the difference between two conditional markets reliable (except for monumentally large decisions), and also that any prediction market subsidies in futarchy won't be well targeted.
Hanson points out that futarchy could authorize predictocracy-like decision making for particular decisions, and vice versa, and so he argues that we should pick the system that would make better decisions on the largest issues. But I worry that the caveats about conditional markets suggest that futarchy might not be the best vehicle for determining whether predictocracy should be used for particular realms of decision making. It would work only if large enough realms were being carved out to make a meaningful impact on GDP+.
Hanson makes some strong points in favor of futarchy. "Democracy today suffers from enormous errors regarding estimates of policy consequences, i.e., of passing particular bills," he points out. Predictocracy reduces the effects of the errors, since evaluations can be made years after a policy is enacted, but ex post evaluators in predictocracy might make some systematic errors that prediction market traders in futarchy would fix.
Futarchy, however, introduces another type of error, the danger that the legislature will not do a good job of defining GDP+, as Hanson acknowledges. It's not a priori clear which would be worse -- errors by the legislature in developing a formula for GDP+, or errors by ex post evaluators in determining whether a particular policy has increased or decreased general welfare. It probably depends to some extent on the quality of our legislature and the quality of our average ex post judges.
Ultimately, the question reduces to this: Suppose all you knew about a policy was that (a) one prediction market forecast that it would increase a measure of GDP+ devised by the legislature; and (b) another prediction market forecast that people some years later would conclude that this policy was a bad idea.
I would tentatively suppose that the participants in market (b) recognized some limitation of GDP+ that would be apparent after enactment of the policy. Robin would guess that the participants in market (b) anticipated that the ex post evaluators would fail to identify some actual policy consequence of the policy.
Given my views on this question, and the challenges of using futarchy for relatively small decisions, I would prefer predictocracy. Most readers who have followed the argument so far probably prefer traditional forms of republican government -- and I do too, because of transition problems and uncertaintty.
Ultimately, I believe that both markets forecasting particular consequences of potential government decisions and normative markets forecasting ex post assessments of policies could be useful tools within traditional republican governance.
The Biggest Prediction Market of the Year:
The graph is of the last hour of the Giants' season, as viewed through the Tradesports.com prediction market.
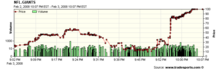
This Giants fan thanks Eugene and the rest of the Conspiracy for letting me guest-blog the past week about my book Predictocracy and about prediction markets. I hope that I have persuaded you at least that prediction markets have the potential to be useful inputs into our public and private decision-making processes.
Meanwhile, I hope to have encouraged at least a few of you to consider writing about the possible use of prediction markets in decision-making institutions. My future research will mostly take me away from prediction markets, but I would be happy to chat with anybody (including, of course, law students) who are interested in doing work in this area. I have many further ideas for applications, experiments, and analyses that did not make it into the book, and would enjoy hearing about your own ideas.
|
|